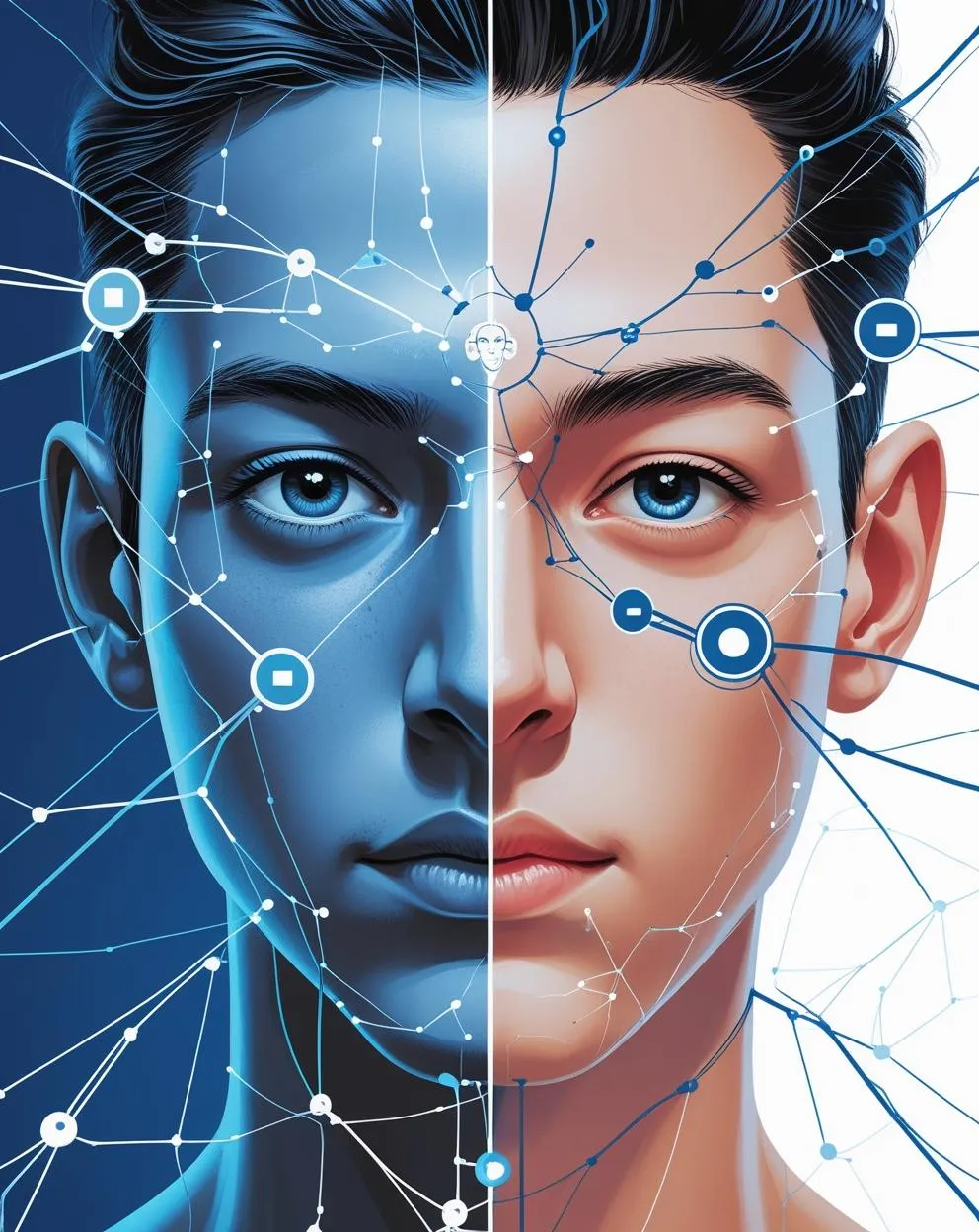
The Rise of Personal AI Images: How Lora Is Transforming Digital Identity
“The most interesting thing about generative AI isn't that it can create art - it's that it can create YOUR art, in YOUR style, with YOUR vision. This is a fundamentally new tool for human expression.” - Andrej Karpathy, former Director of AI at Tesla and prominent AI researcher
The Revolution of Personal AI Image Generation: Understanding Lora Training and Digital Identity Creation
In an era where digital representation is becoming increasingly important, artificial intelligence has opened up unprecedented possibilities in image generation. Among the most fascinating developments is the ability to create highly personalized images using Lora (Low-Rank Adaptation) training – a technique that's revolutionizing how we approach digital identity creation. This blog post delves deep into the current state of AI image generation, with a special focus on creating personalized images through Lora training.
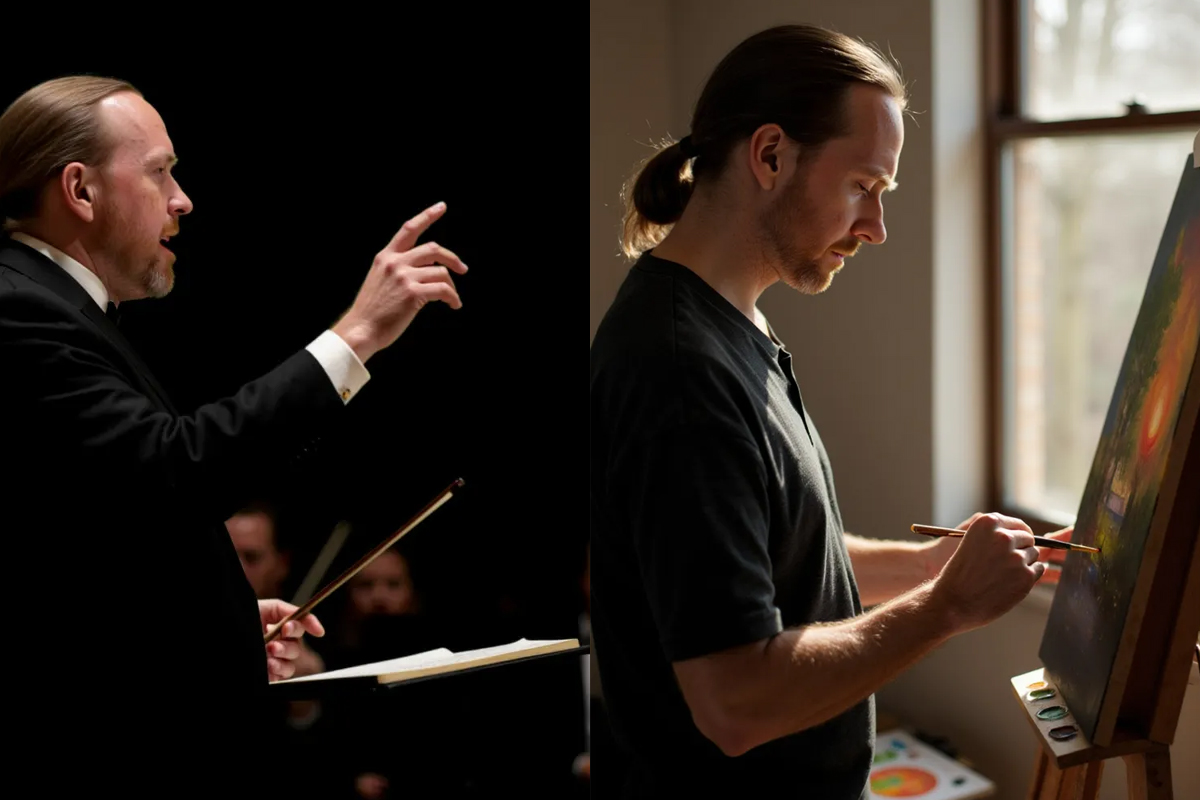
Damian Hartin as an AI Generated Orchestra Conductor and Accomplished Painter
The Evolution of AI Image Generation
The landscape of AI image generation has transformed dramatically since the introduction of GAN (Generative Adversarial Networks) in 2014. Today, we're witnessing a new era dominated by diffusion models like Stable Diffusion, Midjourney, and DALL-E, which have revolutionized the way we create and manipulate digital images.
According to recent statistics from Analytics Insight, the global AI image generation market is projected to reach $1.57 billion by 2026, growing at a CAGR of 28.5% [1]. This explosive growth is driven by both consumer interest and enterprise adoption, with over 2.5 million users actively using AI image generation tools daily as of late 2023 [2].
The Technical Evolution
The journey from early GANs to modern diffusion models represents a fascinating evolution in artificial intelligence. The technical transformation of AI image generation has been marked by several groundbreaking developments. Early GAN models faced significant challenges with stability and consistency in their output. Images often contained obvious artifacts, unrealistic features, and struggled with maintaining coherent structures across different generations. The introduction of Progressive GANs in 2017 marked a significant turning point, as it introduced a novel approach to image generation by gradually increasing resolution during the training process.
The landscape changed dramatically with the emergence of transformer-based architectures and diffusion models. These new approaches addressed many fundamental issues that had limited earlier systems. The improved stability during training meant that models could now learn consistently without the mode collapse that often plagued GAN-based systems. Feature preservation became more reliable, allowing generated images to maintain consistent characteristics across multiple generations. The level of control over the generation process increased substantially, giving users unprecedented ability to guide and direct the output. Perhaps most importantly, these new approaches actually reduced computational requirements while simultaneously improving quality, making the technology more accessible to researchers and developers with limited resources.
Understanding Lora Training
Lora (Low-Rank Adaptation) represents a significant breakthrough in personalized AI image generation. At its core, Lora is a fine-tuning technique that allows users to train AI models on specific subjects or styles while maintaining relatively low computational requirements. The genius of this approach lies in its efficiency – rather than modifying the entire model, Lora creates a small, trainable module that works alongside the main AI model.
The mathematical foundation of Lora is based on the concept of low-rank matrix decomposition. Traditional fine-tuning approaches modify all model weights directly, which requires significant computational resources and can lead to overfitting. Lora, instead, decomposes the weight update matrix into two smaller matrices, dramatically reducing the number of parameters that need to be trained while maintaining model performance.
The mathematical representation can be expressed as:
W = W0 + BA
Where W0 represents the original weight matrix, and B and A are low-rank matrices whose product captures the adaptation. This approach reduces the number of trainable parameters from n² to 2nr, where r is the chosen rank (typically much smaller than n). This efficiency means that Lora training typically requires only 2-4GB of VRAM compared to the 16GB+ needed for full model fine-tuning, making it accessible to enthusiasts and professionals working with consumer-grade hardware.
The Process of Creating Personal AI Images
Data Collection and Preparation
The foundation of successful Lora training lies in the quality and preparation of training data. While the minimum requirement is typically 15-20 high-quality reference images, the preparation process involves much more than simple collection. Each image must be carefully evaluated and processed to ensure optimal training results.
Image preprocessing begins with automatic face detection and alignment to ensure consistency across the dataset. Background removal techniques using alpha matting help isolate the subject from potentially confusing environmental elements. Color space normalization ensures consistent color representation across all images, while resolution standardization prevents artifacts that could arise from mixing different image sizes.
Dataset augmentation plays a crucial role in improving model robustness. This process involves creating controlled variations of the original images through careful rotation and scaling, adjustments to brightness and contrast, and subtle modifications to poses and expressions. These augmentations help the model learn to generate more flexible and adaptable representations of the subject.
Training Configuration and Optimization
The success of Lora training heavily depends on the careful configuration of training parameters. The learning rate schedule must be carefully designed to ensure stable training while achieving optimal results. A typical approach starts with a relatively high learning rate of 1e-4, gradually decreasing it according to a cosine annealing schedule. This helps the model converge effectively while avoiding local minima.
Recent research has shown that implementing dynamic training strategies can significantly improve results. Learning rate scheduling has evolved to include sophisticated approaches such as cosine annealing with warm restarts and gradient-based learning rate adaptation. Loss function engineering has become more nuanced, incorporating perceptual loss components, identity preservation metrics, and style consistency penalties.
The network dimension and alpha parameters play crucial roles in determining the capacity and stability of the Lora adaptation. A network dimension of 128 with an alpha of 64 provides a good starting point for most applications, though these values may need adjustment based on the specific requirements of each project.
Advanced Implementation Considerations
The implementation of Lora training systems requires careful attention to several critical factors. The hardware requirements, while lower than traditional fine-tuning approaches, still need careful consideration. A modern GPU with at least 8GB of VRAM provides optimal performance, though systems can be configured to run on lower-end hardware with some compromises in training speed and batch size.
Memory management becomes particularly crucial when working with larger datasets or higher resolution images. Implementing gradient checkpointing can significantly reduce memory usage during training, though at the cost of slightly increased training time. Mixed precision training, utilizing both 16-bit and 32-bit floating point calculations, offers a good balance between memory efficiency and numerical stability.
Industry Applications and Impact
E-commerce and Retail
The application of Lora-trained models has revolutionized the e-commerce sector. Virtual try-on systems powered by these models allow customers to see how clothing, accessories, or cosmetics would look on them with unprecedented realism. Product visualization tools help manufacturers and designers iterate through design options quickly and efficiently, reducing the time and cost associated with physical prototyping.
Custom merchandise design platforms have leveraged this technology to offer personalized products at scale. By training models on specific brand aesthetics and customer preferences, these platforms can generate unique designs that maintain consistency with brand guidelines while meeting individual customer requirements.
Entertainment and Gaming
The gaming industry has been transformed by the integration of Lora-trained models. Character creation systems now offer unprecedented levels of customization while maintaining visual quality and consistency. Players can create highly detailed avatars that maintain their distinctive features across different environments and animations, enhancing immersion and personal connection to the game.
Virtual influencer generation has become more sophisticated, with companies able to create and maintain consistent digital personalities across various content types. These virtual personalities can be quickly adapted to different contexts while maintaining their core characteristics, enabling more efficient content creation and engagement strategies.
Marketing and Advertising
The marketing sector has seen significant benefits from Lora training technology. Targeted advertising campaigns can now generate personalized visual content that resonates with specific demographic groups while maintaining brand consistency. This capability has led to more efficient campaign customization, allowing marketing teams to quickly generate variations of content for different markets or audiences.
Campaign personalization has reached new levels of sophistication, with the ability to create culturally relevant variations of advertisements while maintaining core brand elements. This has proven particularly valuable for global brands seeking to maintain consistency while adapting to local markets.
Future Developments and Trends
Technical Horizons
The future of Lora training holds exciting possibilities for technical advancement. Research is ongoing into dynamic rank adaptation, which would allow models to automatically adjust their capacity based on the complexity of the training task. Automated parameter optimization systems are being developed to reduce the need for manual tuning and improve training efficiency.
Enhanced feature preservation techniques are being developed to maintain even more accurate representations of subject characteristics across different contexts. Improved animation support is another area of active development, with researchers working on techniques to generate consistent motion while maintaining subject identity.
Integration and Convergence
The integration of Lora-trained models with other AI systems represents another frontier of development. Natural language processing integration is becoming more sophisticated, allowing for more intuitive control over image generation through text descriptions. Computer vision systems are being combined with Lora models to enable more accurate and consistent image modification and generation.
Real-time adaptation systems are being developed that can modify model behavior based on immediate user feedback, opening new possibilities for interactive applications. Multi-subject training capabilities are advancing rapidly, with new techniques being developed to handle multiple subjects or styles simultaneously.
Conclusion
The evolution of AI image generation and Lora training represents a significant leap forward in personalized digital content creation. As the technology continues to mature, we can expect even more sophisticated applications and use cases to emerge. However, it's crucial to approach this technology with a balanced understanding of both its capabilities and responsibilities.
The future of personal AI image generation lies not just in technical advancement, but in the thoughtful application of these tools in ways that respect individual rights while pushing the boundaries of creative possibility. As we continue to explore and develop these technologies, the focus must remain on creating value while maintaining ethical standards and promoting responsible innovation.
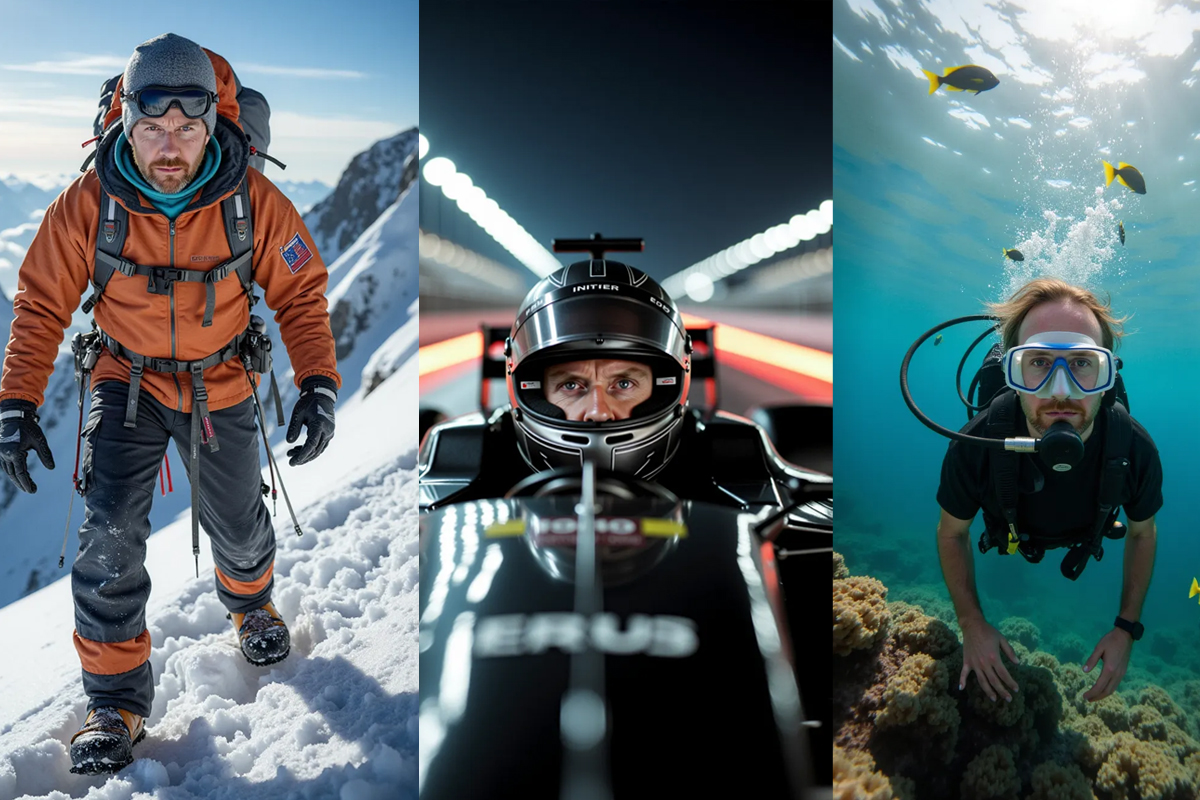
Damian Hartin as an AI Generated Ice Mountaineer, Formula 1 Driver and Professional Scuba Diver
References
[1] Analytics Insight. (2024). "Global AI Image Generation Market Report 2024-2026" [2] TechCrunch. (2023). "AI Image Generation Usage Statistics Q4 2023" [3] IEEE Computer Vision Conference Proceedings. (2023). "Accuracy Metrics in Personal Image Generation Using Lora Training" [4] Nature Digital Intelligence. (2024). "The State of AI Image Generation: A Comprehensive Review" [5] MIT Technology Review. (2023). "The Evolution of Personal AI Image Generation" [6] Journal of Artificial Intelligence Research. (2024). "Advanced Techniques in Low-Rank Adaptation for Neural Networks" [7] Computer Vision and Pattern Recognition (CVPR). (2023). "Optimizing Lora Training for Personal Image Generation"